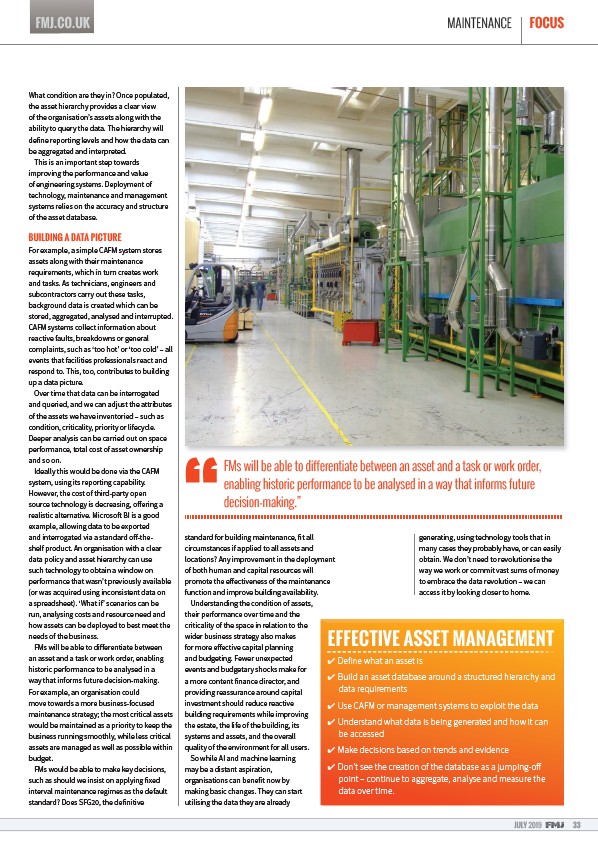
FMJ.CO.UK MAINTENANCE FOCUS
)0s will be able to diff erentiate between an asset and a task or work order,
enabling historic performance to be analysed in a way that informs future
decision-making.”
generating, using technology tools that in
many cases they probably have, or can easily
obtain. We don’t need to revolutionise the
way we work or commit vast sums of money
to embrace the data revolution – we can
access it by looking closer to home.
JULY 2019 33
What condition are they in? Once populated,
the asset hierarchy provides a clear view
of the organisation’s assets along with the
ability to query the data. The hierarchy will
define reporting levels and how the data can
be aggregated and interpreted.
This is an important step towards
improving the performance and value
of engineering systems. Deployment of
technology, maintenance and management
systems relies on the accuracy and structure
of the asset database.
BUILDING A DATA PICTURE
For example, a simple CAFM system stores
assets along with their maintenance
requirements, which in turn creates work
and tasks. As technicians, engineers and
subcontractors carry out these tasks,
background data is created which can be
stored, aggregated, analysed and interrupted.
CAFM systems collect information about
reactive faults, breakdowns or general
complaints, such as ‘too hot’ or ‘too cold’ – all
events that facilities professionals react and
respond to. This, too, contributes to building
up a data picture.
Over time that data can be interrogated
and queried, and we can adjust the attributes
of the assets we have inventoried – such as
condition, criticality, priority or lifecycle.
Deeper analysis can be carried out on space
performance, total cost of asset ownership
and so on.
Ideally this would be done via the CAFM
system, using its reporting capability.
However, the cost of third-party open
source technology is decreasing, o ering a
realistic alternative. Microso BI is a good
example, allowing data to be exported
and interrogated via a standard o -theshelf
product. An organisation with a clear
data policy and asset hierarchy can use
such technology to obtain a window on
performance that wasn’t previously available
(or was acquired using inconsistent data on
a spreadsheet). ‘What if’ scenarios can be
run, analysing costs and resource need and
how assets can be deployed to best meet the
needs of the business.
FMs will be able to di erentiate between
an asset and a task or work order, enabling
historic performance to be analysed in a
way that informs future decision-making.
For example, an organisation could
move towards a more business-focused
maintenance strategy; the most critical assets
would be maintained as a priority to keep the
business running smoothly, while less critical
assets are managed as well as possible within
budget.
FMs would be able to make key decisions,
such as should we insist on applying fixed
interval maintenance regimes as the default
standard? Does SFG20, the definitive
standard for building maintenance, fit all
circumstances if applied to all assets and
locations? Any improvement in the deployment
of both human and capital resources will
promote the e ectiveness of the maintenance
function and improve building availability.
Understanding the condition of assets,
their performance over time and the
criticality of the space in relation to the
wider business strategy also makes
for more e ective capital planning
and budgeting. Fewer unexpected
events and budgetary shocks make for
a more content finance director, and
providing reassurance around capital
investment should reduce reactive
building requirements while improving
the estate, the life of the building, its
systems and assets, and the overall
quality of the environment for all users.
So while AI and machine learning
may be a distant aspiration,
organisations can benefit now by
making basic changes. They can start
utilising the data they are already
EFFECTIVE ASSET MANAGEMENT
✔ Define what an asset is
✔ Build an asset database around a structured hierarchy and
data requirements
✔ Use CAFM or management systems to exploit the data
✔ Understand what data is being generated and how it can
be accessed
✔ Make decisions based on trends and evidence
✔ Don’t see the creation of the database as a jumping-o
point – continue to aggregate, analyse and measure the
data over time.